فهرست مطالب
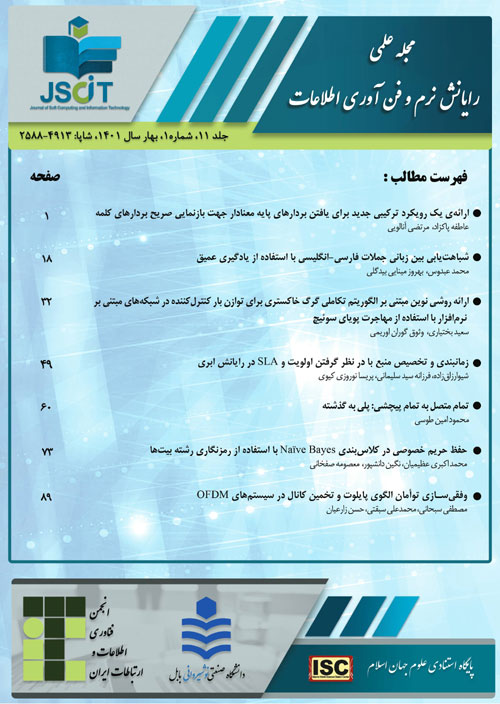
مجله رایانش نرم و فناوری اطلاعات
سال یازدهم شماره 1 (بهار 1401)
- تاریخ انتشار: 1401/04/13
- تعداد عناوین: 7
-
-
صفحات 1-17
هدف اصلی این پژوهش بازنمایی صریح بردارهای معنایی کلمه با ابعاد کم است. برای تولید بردارهای معنایی کلمه صریح، بایستی مسیله ی یافتن تعداد محدودی بردار پایه معنادار به گونه ای حل شود که با کاهش ابعاد بردارهای کلمه افت دقت زیادی ایجاد نشود. ما در این پژوهش یک رویکرد ترکیبی برای یافتن بردارهای پایه معنادار ارایه می کنیم. در ابتدا، N بردار پایه را با روش های پیشنهادی 1-معیار نسبت شباهت کلمه به تکرار کلمه، 2-انتخاب ویژگی مبتنی بر مقایسه ماتریس های فاصله، 3-وز ن دهی دودویی مبتنی بر الگوریتم BPSO بدست می آوریم. سپس، برای بهره گیری از خبرگی روش های 1و 2 به میزان یکسان، نیمی از بردارهای پایه بدست آمده با روش معیار نسبت شباهت کلمه به تکرار کلمه را با نیمی از بردارهای پایه انتخاب شده با روش انتخاب ویژگی، ترکیب کرده و بردارهای پایه ترکیبی اول را بدست می آوریم. در مرحله بعدی، کلمات بافتار مشترک دارای وزن "1" بدست آمده با استفاده از روش BPSO را به بردارهای پایه ترکیبی اول حاصل از روش های نسبت شباهت کلمه به تکرار کلمه و روش انتخاب ویژگی می افزاییم. بنابراین، بردارهای پایه ترکیبی دوم بدست می آیند که معنادار هستند و هر بردار پایه معادل یک کلمه بافتار آگاهی بخش است. لذا بردارهای کلمه صریح تولید شده با استفاده از بردارهای پایه معنادار، تفسیرپذیر هستند. ما رویکرد پیشنهادی را با استفاده از پیکره UkWaC آموزش داده و بر روی وظیفه شباهت کلمه مورد ارزیابی قرار می دهیم. هر دو بردارهای پایه ترکیبی اول و دوم سبب بهبود دقت می گردند. این افزایش دقت در بردارهای پایه ترکیبی اول بیشتر است. نتایج حاصل از ارزیابی بردارهای کلمه صریح بدست آمده با بردارهای پایه اول نشان می دهد که با وجود کاهش ابعاد بردارهای کلمه از 5000 به 1511، ضریب همبستگی اسپیرمن بر روی مجموعه های آزمون MEN، RG-65 و SimLex-999 به ترتیب به میزان 2.47%، 7.39% و 0.52% افزایش می یابد.
کلیدواژگان: بردارهای پایه، بازنمایی بردارهای کلمه، بردارهای کلمه تفسیرپذیر، وزن دهی دودویی، انتخاب ویژگی، وظیفه شباهت کلمه -
صفحات 18-31
شباهت یابی معنایی متون یکی از زیرشاخه های پردازش زبان طبیعی محسوب می شود که در چند سال اخیر تحقیقات گسترده ای را به خود معطوف کرده است. سنجش تشابه معنایی بین کلمات یا اصطلاحات، جملات، پاراگراف و اسناد، نقش مهمی در پردازش زبان طبیعی و زبان شناسی رایانشی ایفا می کند. شباهت یابی معنایی متون در سامانه های پرسش و پاسخ، کشف تقلب، ترجمه ماشینی، بازیابی اطلاعات و نظیر آن کاربرد دارد. منظور از شباهت یابی معنایی، محاسبه میزان شباهت معنایی بین دو سند متنی، پاراگراف یا جمله می باشد که به دو صورت تک زبانه و چندزبانه مطرح است. در این مقاله با استفاد از پیکره موازی میزان، برای اولین بار مدل بین زبانی شباهت معنایی جملات فارسی-انگلیسی را ارایه داده و در ادامه مدل خود را با مدل برت چندزبانه مورد آزمون و مقایسه قرار دادیم. نتایج حاکی از آن است که با استفاده از پیکره های موازی می توان کیفیت تعبیه جملات را در دو زبان مختلف بهبود بخشید. در روش پیشنهادی، معیار همبستگی پیرسون بر اساس شباهت کسینوسی بین بردارهای معنایی حاصل از برت چندزبانه از 65 درصد به 73.77 درصد افزایش یافته است. روش پیشنهادی بر جفت زبان عربی-انگلیسی نیز مورد آزمون قرار گرفت که نتایج حاصله بیانگر برتری روش پیشنهادی نسبت به برت چند زبانه است.
کلیدواژگان: پردازش زبان طبیعی، شباهت معنایی، بین زبانی، یادگیری عمیق -
صفحات 32-48شبکه مبتنی بر نرم افزار (SDN) در بسیاری از سناریوهای مدرن شبکه به مدلی محبوب برای کنترل و مدیریت متمرکز تبدیل شده است. بااین حال، برای مراکز داده بزرگ با صدها هزار سرور و چندین هزار سوییچ، حالت کنترل کننده تک باعث می شود سیستم از عدم مقیاس پذیری و قابلیت اطمینان رنج ببرد. استفاده از معماری توزیع شده می تواند عملکرد سیستم را بهبود بخشد، اما محدودیت اصلی این کار نگاشت ایستا بین سوییچ و کنترل کننده است که ممکن است منجر به عدم تعادل بار در کنترل کننده ها شود. استفاده از چندین کنترل کننده توزیع شده در SDN برای بهبود مقیاس پذیری و قابلیت اطمینان، جایی که هر کنترل کننده یک بخش ثابت از شبکه را مدیریت می کند، استفاده شده است. درروش پیشنهادی، یک کنترل کننده متعادل (بالکن)، به عنوان طرحی برای انتقال پویای سوییچ SDN برای دستیابی به تعادل بار بین کنترل کننده های SDN با هزینه مهاجرت کم به کار گرفته شده است. تصمیم گیری برای مهاجرت توسط نظارت بر اساس ترافیک شبکه انجام می شود. برای تعادل بار در شبکه مبتنی بر نرم افزار، از یک استراتژی انتقال سوییچ مرحله ای استفاده می شود و کنترل کننده هدف توسط الگوریتم گرگ خاکستری انتخاب می گردد. نتایج نشان می دهد که زمان پاسخ روش پیشنهادی 13% بهتر از روش بالکن است. همچنین با افزایش نرخ ارسال به 8.33%، توان عملیاتی کنترل کننده در مقایسه با روش بالکن بهبود می یابد. لذا، تعادل بار روش پیشنهادی عملکرد بهتری نسبت به کارهای پیشین دارد.کلیدواژگان: شبکه مبتنی بر نرم افزار، مهاجرت سوئیچ، تعادل بار، الگوریتم گرگ خاکستری
-
صفحات 49-59
در چند دهه ی اخیر با محبوبیت و کاربردی شدن رایانش ابری، تغییرات قابل توجهی در ارتباطات و فناوری ها شکل گرفته است. به صورتی که بیشتر سرویس دهندگان خدمات در سطح سرویس، در حال ارایه برنامه ها و توسعه زیرساخت های سخت افزاری خود بعنوان بهبود خدمات هستند. از آنجایی که رایانش ابری منابع مختلف را در اختیار این سرویس دهندگان قرار می دهد طبعا هزینه دسترسی، سرعت و دیگر پارامترهای مهم منجر به استقبال چشم گیر شده است; اما نکته ی مهم با توجه به ازدیاد و حجم درخواست های ذینفعان منجر به بروز چالش هایی در ارایه خدمات در سطح سرویس شده است. لذا زمانبندی و تخصیص منابع به درخواست های انجام شده با افق هزینه و زمان اتمام کار پایین، یک ضرورت شده است و سرویس دهندگان به دنبال دریافت بهترین سرویس ممکن با کارایی بالا هستند. این امر منجر به تحقیقات گسترده در این حوزه شده است. روش پیشنهادی مدلی برای زمانبندی و تخصیص منابع با در نظر گرفتن اولویت و توافق نامه سطح سرویس در رایانش ابری ارایه شده است. در حقیقت مدل ارایه شده در چند سطح مختلف دسترسی برای محقق کردن هدف اصلی، که زمانبندی و تخصیص بهینه منابع به درخواست ها می باشد، توسعه داده شده است. در روش ارایه شده به کمک الگوریتم نوبت گردشی و تکنیک وزن دهی به درخواست ها و نیز بررسی برخط ماشین های مجازی بهترین منبع ممکن برای تخصیص پیشنهاد و زمانبندی شده است. روش ارایه شده در قالب یک مدل پویا و قابل اجرا به کمک ابزار کلودسیم شبیه سازی و پارامترهای طول زمانبندی، هزینه و سرعت پردازش با چند روش مشابه مقایسه و آنالیز شده است. نتایج بدست آمده از سناریوهای مختلف بیانگر کاهش 8.68 ثانیه در متوسط طول زمانبندی و افزایش2.15 در متوسط سرعت پردازش است. همچنین هزینه پردازش تغییر قابل توجهی نداشته است
کلیدواژگان: رایانش ابری، نخصیص منابع، زمانبندی، توافق نامه سطح سرویس -
صفحات 60-72
در یک دهه ی گذشته شبکه های پیچشی متعددی برای قطعه بندی معنایی تصاویر ابداع شده اند که عملکرد بسیار خوبی در تشخیص و برچسب زنی اشیاء از خود نشان داده اند. عمده ی این شبکه ها متضمن معماری های با اندازه ی بزرگ هستند که توانایی آشکارسازی ده ها یا صدها دسته ی از قبل مشخص را داشته باشند. در بیشتر کاربردها از معماری هایی استفاده می شود که پس از چند لایه ی پیچشی از یک طبقه بند معمول برای طبقه بندی ویژگی های استخراج شده ی شبکه استفاده می شود. در این نوشتار روش تبدیل یک شبکه که به عنوان طبقه بند، دو لایه ی مسطح و چگال (تمام متصل) دارد، به یک شبکه تمام پیچشی بیان شده است. مزیت اصلی این شیوه، قابلیت کارکرد بر روی ورودی های با اندازه متغیر و تولید یک نقشه خروجی به جای یک عدد می باشد که همان مزیت شبکه های تمام پیچشی است. در مدل های جدید حوزه ی یادگیری عمیق عموما از تصاویر آموزشی که در آنها نواحی موردنظر با ماسک مشخص شده اند استفاده می شود، اما در شیوه ی پیشنهادی در این نوشتار فقط تصاویر برچسب دار (مشخص کننده طبقه ی کل تصویر) به شبکه داده می شود. جزییات روش کار در قالب مسیله ی جدید طبقه بندی و شناسایی تابلوهای با رسم الخطهای شکسته نستعلیق و ثلث، شناسایی برگ سالم از مریض سیب (به عنوان مسایل دو کلاسه) و مسیله ی شناسایی ارقام فارسی بیان شده است. به این منظور ابتدا یک شبکه پیچشی با لایه آخر تمام متصل طراحی و بر روی تصاویر مربعی آموزش داده می شود. سپس مدل تمام پیچشی جدیدی بر اساس مدل قبلی تعریف شده و وزنهای مدل قبلی به مدل جدید کپی می شود. تنها تفاوت دو مدل در لایه آخر است، اما مدل جدید قابلیت کار بر روی تصاویر ورودی با هر اندازه را خواهد داشت. نتایج آزمایشات کارایی این شیوه را نشان داده است .
کلیدواژگان: یادگیری عمیق، شبکه های عصبی پیچشی، طبقه بندی تصویر، شناسایی اشیاء، پرسپترون چند لایه -
صفحات 73-88ساخت مدل های کلاس بندی به طور گسترده ای در داده کاوی مورد استفاده قرار می گیرد. از آنجا که برای ساخت مدل ها نیاز به جمع آوری داده است، نگرانی هایی در زمینه ی حریم خصوصی مالکین داده ها وجود دارد. در این مقاله یک طرح ساخت مدل کلاس بندی Naïve Bayes ارایه شده است که با مشارکت مالکین داده ها و بدون نیاز به جمع آوری اصل داده ها، عملیات ساخت مدل را انجام می دهد. این طرح به جای جمع آوری داده ها، با استفاده از رمزنگاری رشته بیت های حاصل از شمارش و بدون افشای داده ها، فرآیند ساخت مدل Naïve Bayes را انجام می دهد. این طرح بدون نیاز به اعتماد به شخص سوم[i] با حداقل تعداد اجرای عملیات رمزنگاری، امکان ساخت مدل را با کارایی مناسب فراهم می کند به طوری که از نظر پیچیدگی زمانی تا 87٪ بهبود در هزینه ی زمانی مشاهده می شود و حافظه ی مصرفی نیز افزایش چندانی نسبت به طرح های دارای عملیات رمزنگاری نداشته است.کلیدواژگان: داده کاوی، کلاس بندی، Naï، ve Bayes، امنیت، حریم خصوصی، محرمانگی
-
صفحات 89-99در سیستم های مخابراتی بی سیم که امروزه کاربرد گسترده ای یافته است، تغییرات فرکانسی و زمانی کانال یکی از مشکلات اساسی است؛ از این رو مساله تخمین کانال و جبران اثرات آن چالشی کلیدی در این سیستم ها محسوب می شود. تکنیک OFDM به دلیل مقاومت در برابر فرکانس گزینی، بهره وری فرکانسی و نرخ ارسال بالا مورد توجه قرار دارد. تخمین کانال بر مبنای پایلوت از پرکاربردترین روش هاست که در بسیاری از سیستم های OFDM از جمله شبکه های موبایل و استانداردهای پخش صدا و ویدیوی دیجیتال استفاده شده است. تعداد و آرایش پایلوت ها در صفحه زمان- فرکانس تاثیر مستقیم در دقت و سرعت فرآیند تخمین کانال مخابراتی دارد. در ارتباطات واقعی از آرایش پایلوت ثابتی استفاده می شود که معمولا با فرض شرایط نوعی برای محوشوندگی کانال طراحی می گردد. این امر منجر به سربار پایلوت غیرضروری در وضعیت خوب کانال (نزدیک به محوشوندگی کند و تخت) و همچنین تخریب عملکرد در بدترین وضعیت کانال می شود. لذا در تحقیقات پیشین، پیشنهاد وفقی سازی آرایش پایلوت ها بر اساس پاسخ کانال مطرح شده است. در واقع یافتن بهترین آرایش پایلوت متناسب با پاسخ کانال، یک مساله بهینه سازی است که رویکرد وفقی به دنبال دستیابی به پاسخ آن است. در این پژوهش روش جدیدی برای وفقی سازی آرایش پایلوت ها بر اساس فیلتر کالمن ارایه و با الگوریتم تخمین کانال ترکیب شده است. بدین ترتیب در روش پیشنهادی، محل پایلوت ها و پاسخ کانال توام با یکدیگر تخمین زده می شود. این روش در شرایط مختلف کانال شبیه سازی شده و عملکرد آن با معیارهای نرخ خطای بیت (BER) و درصد سربار پایلوت ارزیابی گردیده است. نتایج شبیه سازی ها نشان می دهد روش پیشنهادی با درصد سربار پایلوتی کمتر از روش های موجود به عملکردی مشابه دست می یابد که متناظر با افزایش ظرفیت انتقال داده به میزان قابل توجهی است.کلیدواژگان: تخمین کانال، آرایش پایلوت وفقی، سربار پایلوت، فیلتر کالمن، سیستم های چندحاملی فرکانس متعامد
-
Pages 1-17
The main purpose of this study is to represent the semantic word vectors with low dimensions, explicitly. The problem of finding a limited number of meaningful basis vectors for producing explicit semantic word vectors must be solved in such a way that a large accuracy drop is not caused by reducing the dimensions. In this study, we represent a hybrid approach to finding meaningful basis vectors. First, we obtain N basis vectors using the proposed methods 1- The criterion of word similarity-to-word frequency ratio, 2- Feature selection method based on comparison of distance matrices, 3- Binary weighting method based on PSO algorithm. Then, to take advantage of the expertise of methods 1 and 2 to the same extent, we obtain the first combined basis vectors by combining half of the basis vectors obtained by the criterion of word similarity-to-word frequency ratio with half of the basis vectors selected by the feature selection method. In the next step, we obtain the common context words that have a weight "1" as the common basis vectors produced by the binary weighting method. In the next step, we add the common context words with a weight "1" obtained using the BPSO method to the first combined basis vectors obtained from word similarity-to-word frequency ratio and the feature selection methods. Thus, the second combined basis vectors are obtained, which are meaningful, and each basis vector is equivalent to an informative context word. Therefore, the explicit word vectors produced by meaningful basis vectors can be interpreted. We train the proposed approach using the UkWaC corpus and evaluate it using the word similarity task. Both first and second combined basis vectors improve accuracy. The increase in accuracy is greater in the first combined basis vectors. The evaluation results of explicit word vectors obtained with the first basis vectors show that despite the reduction of word vector dimensions from 5000 to 1511, the Spearman correlation coefficient on MEN, RG-65, and SimLex-999 test sets is increased by 2.47%, 7.39%, and 0.52%, respectively.
Keywords: basis vectors, word vector representation, interpretable word vectors, binary weighting, Feature selection, word similarity task -
Pages 18-31
Semantic Textual similarity is one of the subtasks of natural language processing that has attracted extensive rese arch in recent years. Measuring semantic similarity between words, sentences, paragraphs, and documents plays an important role in natural language processing and computational linguistics. Semantic similarity of texts is used in question-answering systems, fraud detection, machine translation, information retrieval and etc. Semantic similarity means calculating the degree of similarity between two textual documents, paragraphs or sentences, which are presented in both monolingual and cross lingual forms. In this article, by using the parallel corpus, for the first time, the cross lingual model of semantic similarity for Persian-English sentences is presented, and then we test and compare our model with the Multilingual BERT model. The results show that by using parallel corpuses, the quality of sentence embedding in two different languages can be improved. Pearson correlation criterion based on cosine similarity between sentence's vector of multilingual Bert has increased from 65% to 73.77% by the proposed method. The proposed method was also tested on the Arabic-English language pair, and the results show that the proposed method is superior to the multilingual Bert.
Keywords: Natural Language Processing, Semantic Similarity, cross lingual, Deep Learning -
Pages 32-48Software Defined Network (SDN) has become a popular model for centralized control and management in many modern network scenarios. However, for large data centers with hundreds of thousands of servers and several thousand switches, a single controller mode causes the system to suffer from lack of scalability and reliability. The use of distributed architecture can improve system performance, but the main limitation of this work is the static mapping between the switch and the controller, which may lead to load imbalances in the controllers. The use of multiple controllers distributed in SDN has been used to improve scalability and reliability, where each controller manages a fixed partition of the network. In the proposed method, a balanced controller (BalCon) is used as a scheme to dynamic migrate the SDN switch to achieve load balance between SDN controllers with low migration cost. The decision to migrate is made by the monitor based on network traffic. To balance load in the software-based network, a staged switch migration strategy is used and the target controller is selected by the gray wolf algorithm. The results show that the response time of the proposed method is 13% better than the BalCon method. Also, by increasing the sending rate to 8.33%, the controller's throughput improves compared to the BalCon method. Therefore, the load balance of the proposed method has a better performance than previous works.Keywords: Software defined network, Switch Migration, Load Balancing, Gray wolf algorithm
-
Pages 49-59
In recent decades, with the popularity and application of cloud computing, significant changes have taken place in communications and technologies. Most service-level service providers are offering applications and developing their own hardware infrastructure as service improvements. Since cloud computing provides different resources to these providers, of course, the cost of access, speed, and other important parameters have led to a significant response, but an important point has given the increase and volume of requests from stakeholders. It has led to challenges in providing services at the service level. Therefore, scheduling and allocating resources to requests made with low-cost horizons and completion time has become a necessity, and service providers and stakeholders seek to receive the best possible service with high efficiency, and this has led to extensive research in this area. In this research, a model for scheduling and resource allocation considering priority and SLA in cloud computing is presented. In fact, the proposed model of several different levels of access has been developed to achieve the main goal of the research, which is the optimal scheduling and allocation of resources to the requests made. In the proposed method, using the RR algorithm and the technique of weighting requests and online review of virtual machines, the best possible source for proposal allocation and scheduling has been identified. The method presented in the form of a dynamic and executable model with the help of the Cloudsim simulation tool and in terms of Makespan, cost, and speedprocess have been compared and analyzed with several similar methods. The results obtained from the simulation performed by applying different scenarios indicate average processing speed around 2.15, and average Makespan is reduced at 8.68s by new method than similarity methods. Also, the rang of cost has not big change.
Keywords: cloud computing, Resource Allocation, scheduling, Service Level Agreement -
Pages 60-72
In the last decade, several convolutional networks have been developed for the semantic segmentation, which have shown excellent performance in recognizing and labeling objects in images. Most of these networks involve large-scale architectures that can detect tens or hundreds of predefined classes. With the exception of fully convolutional networks, most applications use architectures that, after convolutional layers, use a common classifier to classify the extracted features. In this paper, the method of converting a network, which as classifier, has two flatten and dense layers (fully connected), to a fully convolutional network is described. The main advantage of this method is the ability to work on inputs of variable size and produce an output map instead of a number, which is the advantage of fully convolutional networks. Newer models of the Deep Learning area generally use training images in which areas of interest are determined by masks; but in the proposed method only labeled images are given to the network. The details of the proposed method are expressed in the form of a new problem of classification of boards with calligraphy of Shekasteh-Nastaliq and Suls, and classification of apple leaf diseases (as two-class problems) and the problem of identifying hand written Persian digits. For this purpose, first a convolutional network with the last fully connected layer is designed and trained for square images. Then a new fully convolutional model is defined based on the previous model and the weights of the previous model are fed to the new model. The only difference between the two models is in the last layer, but the new model will be able to work on input images of any size. Experimental results show the efficiency of the proposed approach.
Keywords: Deep Learning, Convolutional Neural Networks, Image classification, object detection, Multi-layer Perceptron -
Pages 73-88The construction of classification models is widely used in data mining. There are concerns about the privacy of data owners because of the need to collect data to build models. In this paper, a Naïve Bayes classification model construction plan is presented, which performs the model construction operation with the participation of data owners and without the need to collect the original data. Instead of collecting data, the scheme uses encryption of bit strings from counting without disclosing the original data to perform the process of creating the Naïve Bayes model. This design allows the model to be built with appropriate performance without the need for trust in a third party with a minimum number of encryptions, so that in terms of time complexity, up to 87% improvement in time cost can be observed. In addition, memory consumption has not increased significantly when compared to designs that use encryption operations.Keywords: Data mining, Classification, Naï, ve Bayes, Security, Privacy Preserving, Privacy
-
Pages 89-99In wireless communications which are widely applicable nowadays, the channel variation over time and frequency is a major problem. Therefore, the channel estimation and compensation are critical challenges in these systems. The OFDM technique is noteworthy for wireless channels due to robustness, high efficiency and consequently high data rate. Pilot-aided channel estimation is a promising approach in many OFDM systems, including mobile networks, digital audio and video broadcasting standards. The number and the arrangement of pilots in the time-frequency grid affect the accuracy and the period of the channel estimation procedure. Practical OFDM systems utilize fixed pilot patterns that are designed based on typical assumptions of the fading channel. Thus, unnecessary pilot overhead is imposed when the channel is close to a flat slow fading channel, and the performance degrades in the worst condition of the channel. To remedy this issue, adaptive pilot arrangement based on the channel response is introduced in the previous researches. Indeed, finding the best pilot arrangement according to the channel response is an optimization problem and the adaptive approach is exploited to find its answer. In this paper, we develop a novel method for adaptive pilot arrangement based on Kalman filter which is combined with the channel estimation algorithm. Therefore, the position of the pilots and the channel response are jointly estimated in the proposed method. This method is simulated for various channel types and its performance is evaluated by bit error rate (BER) and pilot overhead criteria. Simulation results illustrate that the proposed method obtains the same performance as the previous methods by fewer pilots. This achievement leads to significant increase in the capacity of data transfer.Keywords: Channel Estimation, Adaptive pilot pattern, Pilot overhead, Kalman Filter, OFDM