فهرست مطالب
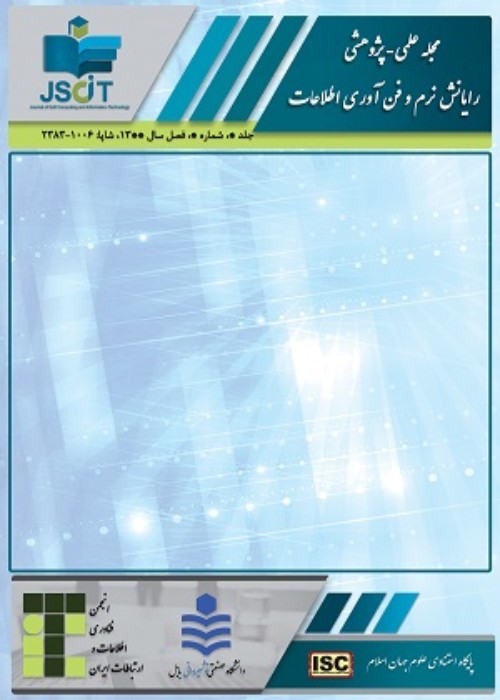
مجله رایانش نرم و فناوری اطلاعات
سال دوازدهم شماره 3 (پاییز 1402)
- تاریخ انتشار: 1402/09/01
- تعداد عناوین: 7
-
-
صفحات 1-16شبکه های اجتماعی یکی از انواع شبکه های پیچیده است. تشخیص جوامع در شبکه های اجتماعی روشی موثر برای بهره گیری از اطلاعات این شبکه ها است که تاکنون الگوریتم های متعددی برای آن ارایه شده است. در این مقاله روش هایی جدید با استفاده از آتوماتاهای یادگیر پیشنهاد شده است که در آنها، یک آتوماتای یادگیر به هر گره شبکه الحاق می شود؛ تعداد اقدام آتوماتاهای یادگیر ثابت و برابر با تخمین تعداد جوامع شبکه است. در هر مرحله، هر کدام از آتوماتاهای یادگیر یک اقدام از مجموعه اقدامات خود را انتخاب می کند. انتخاب هر یک از این اقدام ها به منزله ی انتساب برچسب آن جامعه به گره است. اقدام انتخاب شده توسط هر آتوماتا بر اساس اقدام های انتخابی همسایگانش (بررسی محلی) و/یا جوامع تشخیص داده شده توسط کل روش (بررسی سراسری) ارزیابی می شود. نتیجه ی ارزیابی منجر به صدور پاداش و جریمه برای آتوماتاها می شود. با دریافت پاداش احتمال انتخاب مجدد اقدام انتخابی توسط آتوماتا، یا همان برچسب جامعه، افزایش می یابد و با دریافت جریمه احتمال این اقدام کاهش می یابد. با تکرار الگوریتم، اقدام بهینه مشخص می گردد تا آنجا که با تکرارهای بیشتر هیچ تغییری در برچسب انتخابی آتوماتای متناظر هر گره رخ نمی دهد و درنتیجه جوامع بهینه به عنوان خروجی الگوریتم مشخص می گردند. مقایسه نتایج حاصل از آزمایش های انجام شده، نشان می دهد روش های پیشنهادی نسبت به برخی روش های پیشین عملکرد بهتری را نشان می دهد؛ به خصوص بر اساس معیار NMI که یکی از معیارهای رایج در ارزیابی روش های تشخیص جامعه است.کلیدواژگان: شبکه های اجتماعی، کشف جوامع، آتوماتای یادگیر، معیار شباهت
-
صفحات 17-29یکی از مهم ترین چالش های شبکه های اجتماعی، بحران اعتماد بین کاربران است. یکی از راهکارها برای حل این چالش، اطلاع کاربران از سطح اعتماد کاربران دیگر است. با کمی سازی اعتماد و استفاده از هوش جمعی می توان این راهکار را عملی کرد. مدل های مختلفی برای محاسبه اعتماد در شبکه های اجتماعی با استفاده از فرمول های ریاضی و بدون در نظر گرفتن تغییرات دایمی اعتماد کاربران بر اساس رویدادهای مختلف ارایه شده است. با این حال، ارایه روشی که بتواند انواع مختلفی از سناریوها و مفروضات را مدل سازی کرده و سطح اعتماد هر کاربر را در هر کدام از حالت ها محاسبه و ارزیابی کند، موضوع بسیار مهمی است. در این مقاله از صورت بندی گرافیکی شبکه های پتری رنگی برای مدل سازی اعتماد و بی اعتمادی بین کاربران در شبکه های اجتماعی و ارزیابی تاثیر رویدادهای مختلف بر تغییر سطح اعتماد هر کاربر در طول زمان استفاده شده است. همچنین در این مقاله، الگوریتمی برای مشخص کردن میزان تاثیرگذاری هر کاربر بر سطح اعتماد کاربر دیگر ارایه شده است تا این اثرگذاری بین کاربران یکسان نباشد. روش ارایه شده این قابلیت را دارد که برای هر تعداد کاربر مدل سازی را انجام داده و صرفا با تغییر مقادیر پارامترهای مدل، سناریوهای مختلفی تعریف کرده و سطح اعتماد هر کاربر را هر سناریو ارزیابی کند. به همین دلیل، روش مدل سازی ارایه شده، که نتایج مدل های آن با شبیه ساز OMNeT++ و تحلیل آماری اعتبارسنجی می شود، می تواند به عنوان شبیه ساز سطح اعتماد کاربران در شبکه های اجتماعی مورد استفاده قرار گیرد.کلیدواژگان: شبکه های اجتماعی، مدل سازی اعتماد و بی اعتمادی، ارزیابی سطح اعتماد، شبکه های پتری رنگی
-
صفحات 30-44
یکی از مهمترین مسایل در شبکه های اجتماعی حجم بالای شایعاتی است که توسط عوامل انسانی و یا ماشینی منتشر می شوند. در چنین شرایطی، تشخیص خودکار شایعات برای ایمن نگهداشتن افکار عمومی در برابر خطرات بالقوه آنها؛ از اهمیت بالایی برخوردار است. در این پژوهش، با استفاده از تکنیکهای یادگیری عمیق یک راهکار جدید برای تشخیص خودکار شایعات مرتبط با بیماریهای همه گیر در شبکه های اجتماعی ارایه شده است. در روش پیشنهادی، ابتدا محتوای پیامهای موجود برای پردازش در گامهای بعدی آماده سازی میشوند. همچنین از قالب ماتریس وزنی برای توصیف خصوصیات محتوایی استفاده شده است. سپس در گام دوم روش پیشنهادی، از شبکه عصبی کانولوشن به منظور استخراج مجموعه ویژگیهای مناسب از ماتریس خصوصیات حاصل از گام قبل استفاده میشود. بدین ترتیب، ماتریس خصوصیات محتوایی به عنوان ورودی شبکه عصبی عمیق بکار میرود و مقادیر وزنی به دست آمده در آخرین لایه تماما متصل این شبکه عصبی به عنوان ویژگی های استخراج شده از آن مورد استفاده قرار میگیرد. درنهایت، از تجمیع چند طبقه بند دودویی به منظور تشخیص شایعات و طبقهبندی ویژگیهای استخراج شده از طریق شبکه عصبی کانولوشن استفاده میشود. بدین منظور، ویژگیهای استخراج شده به صورت همزمان توسط چندین مدل یادگیری پردازش شده و خروجی نهایی سیستم پیشنهادی از طریق رایگیری خروجیهای این سه الگوریتم تعیین میشود. نتایج حاصل از این تحقیق نشان میدهد که با استفاده از روش پیشنهادی میتوان شایعات را با میانگین دقت 98.8 درصد تشخیص داد که نشان از بهبود حداقل 2.4 درصدی دقت تشخیص نسبت به روش های پیشین دارد.
کلیدواژگان: شبکه اجتماعی، مدل انتشار شایعه، تشخیص شایعه، یادگیری عمیق -
صفحات 45-57سامانه های پرسش وپاسخ به عنوان یکی از مهمترین سامانه های هوشمند توانایی پاسخگویی آنی و صریح به پرسش های ورودی را دارند. یکی از چالش های جدید این سامانه ها، قدرت پاسخگویی به پرسش های پیچیده چندگامی است که نیازمند جمع آوری اطلاعات از چندین سند است. در این مقاله یک روش برای حل چالش پاسخدهی به پرسش های پیچیده چندگامی ارایه می گردد. در این روش، ابتدا در یک فرایند دومرحله ای، اسناد مرتبط با پرسش بازیابی می شوند. سپس برای تسهیل در پاسخ دهی به پرسش، نسبت به استخراج دانش از اسناد بازیابی شده و بازنمایی آن در قالب یک گراف اقدام می گردد، در نهایت و برای یافتن پاسخ پرسش، استدلال روی گراف با ترکیب شبکه عصبی گرافی نامتجانس و مبدل انجام می پذیرد.به منظور بررسی میزان کارآمدی روش پیشنهادی، این روش و سایر کارهای مرتبط بر روی مجموعه داده دامنه باز هات پات کیوای مورد آزمایش قرار گرفته است. نتایج بدست آمده در پاسخدهی به پرسش بر مبنای معیار اف وان و تطبیق دقیق به ترتیب مقادیر 86٫51 و 78٫71 گزارش شده است که نشانگر برتری این روش نسبت به سایرکارهای مشابه به خود است.کلیدواژگان: سامانه های پرسش و پاسخ، پرسش پیچیده چندگامی، درک مطلب ماشینی، شبکه عصبی گرافی نامتجانس، مبدل ها
-
صفحات 58-73
استخراج جوامع، از موضوعات چالش برانگیز و مهم در حوزه تحلیل شبکه های اجتماعی است. مکانیسم تشخیص جوامعی که به طور همزمان نیازهای توپولوژیکی و معنایی را برآورده سازد، اهمیت بیشتری پیدا کرده است. اکثر مطالعات موجود بر روی ساختار شبکه، بدون توجه به ویژگیهای معنایی، تمرکز دارند. حال اینکه، معنا و محتوای اجتماعی حاوی دادههای ارزشمندی در مورد علایق، نگرانیها و احساسات افراد است. راهحلهای معنایی محض، منجر به از دست رفتن اطلاعات توپولوژیکی ارزشمند شبکه میشوند. روشهای ادغامی موجود، ساختار و معنا را به صورت بنیادی و اساسی ترکیب نمیکنند. اغلب این الگوریتمها به علت ماهیت طراحی، به یکی از روشهای مذکور متمایل بوده و عملکرد محدودی دارند. در این مقاله یک روش مبتنی بر منطق فازی جهت شناسایی جوامع ارایه میشود. ابتدا معنا و ساختار در یک شبکه مشترک ترکیب و سپس جوامع استخراج میگردند. مجموعهای از آزمایش های شبیه سازی جهت ارزیابی عملکرد روش پیشنهادی در مقایسه با سایر الگوریتمهای موجود در سه زمینه ساختاری، معنایی و ادغامی با استفاده از مجموعه داده های مصنوعی و طبیعی در اندازه های مختلف گره ها، یالها، ویژگی ها انجام شده است. نتایج حاصل نشان می دهند، روش پیشنهادی در رابطه با انسجام شبکه و ویژگی های گره ها به طور موثری عمل می کند.
کلیدواژگان: شبکه های پیچیده پویا، تحلیل شبکه های اجتماعی، شناسایی جوامع، معنا، استنتاج فازی -
صفحات 74-84روش های آماری مکان یابی خطا در نرم افزار وابستگی زیادی به داده های ورودی برنامه داشته و با تغییرات داده ها دچار ناپایداری می شوند. از این رو، تولید داده آزمون مناسب نقش کلیدی در کیفیت فرآیند مکان یابی خطای نرم افزار ایفاء می کند. در این مقاله، روشی برای بهبود مکان یابی خطا با تولید داده های آزمون جدید ارایه می شود. مجموعه آزمون به صورت کمینه و هدفمند، جهت تعیین شاخه خطادار و پس از آن جملات مظنون به خطای درون شاخه، تولید می گردد. ابتدا، محدوده جملات مظنون به خطا در یک مسیر اجرایی مشخص می شود. برای این کار، در مسیر اجرایی خطادار، شرط ها از انتها به ابتدا نقیض شده و با استفاده از حل کننده Z3 داده آزمون برای مسیر مورد نظر ایجاد می گردد. سپس، برنامه مجددا با داده آزمون های به دست آمده توسط فن اجرای نمادین پویا اجرا می شود. با توجه به موفق و یا ناموفق بودن اجرا، مشخص می کنیم که کدام شاخه مظنون به خطا است. بدین ترتیب، محدوده جملات برای اعمال روش علی-آماری به حداقل ممکن می رسد. ارزیابی روش پیشنهادی روی چهار پروژه از مجموعه محک Defect4J، انجام شده است. نتایج نشان دهنده کشف %75 از خطاها با بررسی حداکثر یک درصد از کد این برنامه ها است که در مقایسه با کارهای موجود %98/17 بهبود دارد. همچنین، متوسط جملات مورد بررسی جهت کشف خطا، در بدترین حالت به میزان %78/16 کاهش داشته است.کلیدواژگان: اشاشکال زدایی، مکان یابی خطای نرم افزار، تحلیل علی-آماری، تولید داده آزمون، اجرای نمادین پویا
-
صفحات 85-98حملات سیبیل به طور فزاینده ای در شبکه های اجتماعی در حال رشد و گسترش است. یک کاربر مخرب با هویت جعلی که از آن تحت عنوان حمله سیبیل یاد می شود، می تواند تعداد زیادی حساب جعلی برای تولید هرزنامه، جعل هویت سایر کاربران، کلاهبرداری و دسترسی به بسیاری از اطلاعات کاربران قانونی ایجاد کند. به دلایل امنیتی، چنین حساب های جعلی باید شناسایی و غیرفعال شوند. روش های شناسایی مختلفی برای مقابله با حساب های جعلی پیشنهاد شده است. با این حال، بیشتر این روش ها حساب های جعلی را یا با استفاده از گراف های ساختاری اجتماعی شناسایی می کنند که منجر به عملکرد ضعیف می شود و یا از روش های یادگیری ماشین استفاده می شود که دقت پایینی برای شناسایی حملات سیبیل دارند. در این مقاله، یک روش به نام خوشه بندی ترکیبی پیشنهاد شده است که CNRM نامیده شده است. روش پیشنهادی مبتنی بر خوشه بندی می باشد، بدین صورت که با ترکیب روش های مختلف تشخیص اجتماعات؛ یک روش تشخیص اجتماع جدید ارایه شده است. ترکیب این روش ها منجر به دقت بالاتر، نتایج مطمین تر و پایداری بیشتری شده است. روش CNRM بر روی مجموعه داده های جمع آوری شده از توییتر، ردیت، اینستاگرام و فیسبوک ارزیابی شده است. برخلاف سایر رویکردهای مبتنی بر یادگیری ماشین، روش پیشنهادی بر روی سطوح مختلفی از ویژگی های پروفایل کاربران تمرکز می کند. نتایج ارزیابی نشان داده است که روش CNRM گره های سیبیل را با دقت %85.13 تشخیص می دهد.کلیدواژگان: حمله سیبیل، حساب های جعلی، خوشه بندی ترکیبی، تشخیص حساب های جعلی، تشخیص جوامع، CNRM
-
Pages 1-16Social networks are one of the types of complex networks. Identifying communities in social networks is an effective way to use their information, for which several algorithms have been presented so far. In this paper, novel algorithms are designed, in which a learning automaton is attached to each node; The number of actions of learning automata is fixed and equal to the estimate of the number of network communities. At each step, each of the learning automata chooses an action from its set of actions. Choosing any of these actions means assigning the label of that community to the node. The action chosen by each automaton is evaluated based on the chosen actions of its neighbors ((local attention) and/or communities detected by the entire method (global screening). The result of the evaluation leads to generate rewards or punish signal for the automata. By receiving a reward, the probability of re-choosing the chosen action by the automaton, or the community label, increases, and otherwise, by receiving a fine, the probability of this action decreases. By repeating the algorithm, the optimal action is determined as long as no change occurs in the selected label of the corresponding automata of each node with more iterations, and as a result, the optimal communities are determined as the output of the algorithm. The comparison of the results of the experiments shows the effectiveness of the proposed methods in comparison with the previous methods.Keywords: Social Networks, community discovery, Learning Automata, similarity measure
-
Pages 17-29The crisis of trust between users is one of the most important challenges of social networks. One solution to this challenge is for users to know about the trust level of other users. This solution can be implemented by quantifying trust and using collective intelligence. Various models have been presented to calculate trust in social networks using mathematical formulas and without considering the permanent changes in users' trust based on various events. However, providing an approach that can model various types of scenarios and assumptions and calculate and evaluate the trust level of each user is a very important issue. In this paper, the Colored Petri net, a graphical formalism, has been used to model trust and distrust between users in social networks and to evaluate the impact of various events on the change of the trust level of each user over time. Furthermore, an algorithm is proposed in this paper to determine the influence of each user on the trust level of another user, so that this influence is heterogeneous between users. The proposed approach can model any number of users, define different scenarios by simply changing the model parameters' values, and evaluate each user's trust level in each scenario. The proposed modeling approach which the results obtained from its models are validated with the OMNeT++ simulator and statistical analysis can be used as a simulator of users' trust level in social networks.Keywords: Social Networks, Trust, Distrust Modeling, Trust Level Evaluation, Coloured Petri Nets (CPN)
-
Pages 30-44
One of the most important issues in social networks is the high volume of rumors that are spread by human or machine agents. In such situations, automatic detection of rumors to keep public opinion safe from their potential dangers is of great importance. In this research, using deep learning techniques, a new solution for automatically detecting rumors related to epidemic diseases in social networks will be presented. In the proposed method, first the content of existing messages is prepared for processing in the next steps. Also, weight matrix format has been used to describe content characteristics. Then, in the second step of the proposed method, the convolutional neural network is used to extract the set of suitable features from the matrix of features obtained from the previous step. In this way, the matrix of content features is used as the input of the deep neural network, and the weight values obtained in the last fully connected layer of this neural network are used as the features extracted from it. Finally, the aggregation of several binary classifiers is used in order to detect rumors and classify the features extracted through convolutional neural network. For this purpose, the extracted features are simultaneously processed by several learning models and the final output of the proposed system is determined by voting the outputs of these three algorithms. The results of this research show that by using the proposed method, rumors can be detected with an average accuracy of 98.8%, which shows an improvement of at least 2.4% in detection accuracy compared to the previous methods.
Keywords: social network, rumor propagation model, Rumor detection, deep learning.&lrm -
Pages 45-57Question Answering Systems (QAS) are one of the most important intelligent systems that have the ability to provide instant and explicit answers to input questions. One of the new challenges of these systems is the ability to answer multi-hop complex questions that require information collection from multiple documents. In this paper, a method is proposed to solve the challenge of answering multi-hop complex questions. In this method, first, documents related to the question are retrieved in a two-step process. Then, to facilitate answering the question, knowledge is extracted from the retrieved documents and represented in the form of a graph. Finally, to find the answer to the question, reasoning is performed on the graph using a combination of heterogeneous graph neural network and transformer. To evaluate the effectiveness of the proposed method, this method and other related works have been tested on the HotpotQA open-domain dataset. The results obtained in answering questions based on F1 score and exact match are reported as 86.51 and 78.71 respectively, indicating superiority of this method over similar works.Keywords: Question Answering Systems (QAS), Multi-hop Complex Questions, Machine Reading Comprehension (MRC), Heterogeneous Graph Neural Network (HGNN), Transformers
-
Pages 58-73
Discovering Communities is a fundamental problem in understanding network performance for social network analysis. Traditional community detection methods merely consider the network topologies. Nonetheless, social media contains valuable data about people's interests, concerns, and sentiments, which is not reflected in the structure. The semantic solutions eventuate to the mislay of precious structural information. Most existing combinatorial methods favor one of the mentioned kinds and have limited performance. This paper introduces a 2-phase way based on fuzzy inferences to determine effective social network communities. A series of real-life and synthetic networks have been used to evaluate the proposed method compared with several relevant algorithms. The experimental results proved that the proposed approach performs better in detecting meaningful communities and is more effective concerning network coherence and node attributes.
Keywords: Social Networks Analysis, community detection, Semantic, Fuzzy inference -
Pages 74-84The statistical-based software fault localization approaches highly depend on the program inputs and become unstable as input data changes. Therefore, generating appropriate test data plays an essential role in the quality of the software fault-localization process. This paper presents an approach to improving program fault localization by generating new test data. The minimized test suite determines the faulty execution path and the fault suspiciousness of each statement in the path is generated. First, the suspicious statements in the faulty path are determined. To this aim, the conditions of the faulty execution path are contradicted from the end to the beginning, and test data is created for the desired path using the Z3 solver. Afterward, the program under test is executed with the generated test data using the Concolic testing technique. The fault-suspicious branch is determined depending on the passing or failing of the program execution. As a result, the region of statements for applying the causal-statistical approach is minimized. The proposed approach is evaluated on the four projects in the Defects4J benchmark. The results show that 75% of faults are localized by examining a maximum of 1% of the program's source code. Compared to the related work, the results have improved by 17.98%. Moreover, the mean number of sentences examined for fault localization decreases by 16.78% in the worst case.Keywords: Debugging, Software Fault Localization, Statistical Causal Analysis, Test Data Generation, Concolic Execution
-
Pages 85-98Sybil attacks are increasingly growing and expanding in social networks. A malicious user with a fake identity, known as a Sybil attack, can create a large number of fake accounts to generate spam, impersonate other users, commit fraud, and gain access to many legitimate users' information. For security reasons, such fake accounts should be identified and disabled. Various identification methods have been proposed to deal with fake accounts. However, most of these methods detect fake accounts using social structure graphs, which leads to poor performance, or use machine learning methods, which have low accuracy for identifying Sybil attacks. In this paper, a hybrid clustering method called CRNM is proposed. The proposed method is based on clustering, so that by combining different community detection methods; A new community detection method is presented. The combination of these methods has led to higher accuracy, more reliable results and more stability. The CRNM method has been evaluated on datasets collected from Twitter, Reddit, Instagram and Facebook. Unlike other machine learning-based approaches, the proposed method focuses on different levels of user profile features. The evaluation results have shown that the CRNM method detects Sybil nodes with an accuracy of 85.13%.Keywords: Sibyl attack, Fake Accounts, hybrid clustering, detection of fake accounts, community detection, CNRM