فهرست مطالب
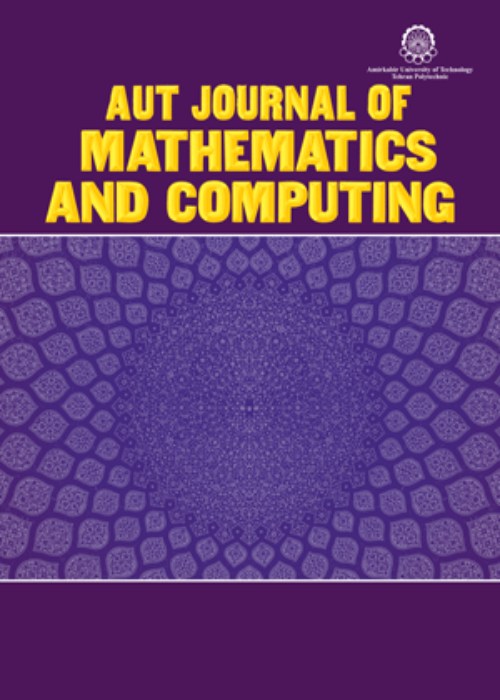
AUT Journal of Mathematics and Computing
Volume:5 Issue: 2, Apr 2024
- تاریخ انتشار: 1403/01/13
- تعداد عناوین: 8
-
-
Pages 81-89Given a group $G$, we define the power graph $\mathcal{P}(G)$ as follows: the vertices are the elements of $G$ and two vertices $x$ and $y$ are joined by an edge if $\langle x \rangle \subseteq \langle y \rangle$ or $\langle y \rangle \subseteq \langle x \rangle$. Obviously the power graph of any group is always connected, because the identity element of the group is adjacent to all other vertices. We consider $\kappa(G)$, the number of spanning trees of the power graph associated with a finite group $G$. In this paper, for a finite group $G$, first we represent some properties of $\mathcal{P}(G)$, then we are going to find some divisors of $\kappa(G)$, and finally we prove that the simple group $A_6\cong L_2(9)$ is uniquely determined by tree-number of its power graph among all finite simple groups.Keywords: power graph, tree-number, simple group
-
Pages 91-104Due to the development of social networks and the Internet of things, we recently have faced with large datasets. High-dimensional data is mixed with redundant and irrelevant features, so the performance of machine learning methods is reduced. Feature selection is a common way to tackle this issue with aiming of choosing a small subset of relevant and non-redundant features. Most of the existing feature selection works are for supervised applications, which assume that the information of class labels is available. While in many real-world applications, it is not possible to provide complete knowledge of class labels. To overcome this shortcoming, an unsupervised feature selection method is proposed in this paper. The proposed method uses the matrix factorization-based regularized self-representation model to weight features based on their importance. Here, we initialize the weights of features based on the correlation among features. Several experiments are performed to evaluate the effectiveness of the proposed method. Then the results are compared with several baselines and state-of-the-art methods, which show the superiority of the proposed method in most cases.Keywords: Unsupervised feature selection, Subspace learning, Self-representation
-
Pages 105-110We continue \cite{sp1 glas} and we discuss approximately left $\varphi$-biprojectivity for $\theta$-Lau product algebras. We give some Banach algebras among the category of $\theta$-Lau product algebras which are not approximately left $\varphi$-biprojective. In fact, some class of matrix algebras under the notion of approximate left $\varphi$-biprojectivity is also discussed here.Keywords: Approximate left $, varphi$-biprojectivity, Approximate left $, varphi$-amenability, $theta$-Lau product
-
Pages 111-123In computer science, a binary decision diagram is a data structure that is used to represent a Boolean function and to consider a compressed representation of relations. This paper considers the notation of T.B.T (total binary truth table), and introduces a novel concept of binary decision (hyper)tree and binary decision (hyper)diagram, directly and in as little time as possible, unlike previous methods. This study proves that every T.B.T corresponds to a binary decision (hyper)tree via minimum Boolean expression and presents some conditions on any given T.B.T for isomorphic binary decision (hyper)trees. Finally, for faster calculations and more complex functions, we offer an algorithm and so Python programming codes such that for any given T.B.T, it introduces a binary decision (hyper)tree.Keywords: Minimum Boolean function, Binary decision hypertree, Binary decision hyperdiagram, Unitor, T.B.T
-
Pages 125-136
The class of homogeneous Siklos space-times is considered from algebraic point of view and the generalized Ricci solitons are completely classified.
Keywords: Siklos space-times, Generalized Ricci solitons, Homogeneous space, Killing vector filed -
Pages 137-143For a real-valued function $f$ on a metric measure space $(X,d,\mu)$ the Hardy-Littlewood centered-ball maximal-function of $f$ is given by the `supremum-norm':$$Mf(x):=\sup_{r>0}\frac{1}{\mu(\mathcal{B}_{x,r})}\int_{\mathcal{B}_{x,r}}|f|d\mu.$$In this note, we replace the supremum-norm on parameters $r$ by $\mathcal{L}_p$-norm with weight $w$ on parameters $r$ and define Hardy-Littlewood integral-function $I_{p,w}f$. It is shown that $I_{p,w}f$ converges pointwise to $Mf$ as $p\to\infty$. Boundedness of the sublinear operator $I_{p,w}$ and continuity of the function $I_{p,w}f$ in case that $X$ is a Lie group, $d$ is a left-invariant metric, and $\mu$ is a left Haar-measure (resp. right Haar-measure) are studied.Keywords: metric measure space, boundedness of sublinear operators
-
Pages 145-159Digital image segmentation plays an important role in noise reduction and pixel clustering for pre-processing of deep learning or feature extraction. The classic Self-Organizing Map (SOM) algorithm is a well-known unsupervised clustering neural network model. This classic method works on continuous data instead of discrete data sets with a widely scattered distribution. The novel SOM(SOM2) modelling solved this problem for the classic, simple tabular discrete data set but not for the digital image data. As the essence of digital image pixels data are different from tabular datasets, we have to look at them differently. This paper proposes exploiting the novel SOM method with a hybrid combination of the fuzzy C-Means and K-means convolution filter as image segmentation and noise reduction with soft and hard segmentation as entropy reduction for natural digital images. The main approach of this paper is the segmentation of image contents for the reduction of noises and saturation pixels by entropy criteria. Based on the resulting paper, the combination of SOM2 with FCM for soft segmentation 47%-and the combination of SOM2 with k-means convolution for hard segmentation 33% can reduce the entropy of the original image on average.Keywords: Image segmentation, self-organizing map, Fuzzy C-Means, K-Means, Entropy-reduction
-
Pages 161-187The Conway group $Co_{1}$ is one of the $26$ sporadic simple groups. It is the largest of the three Conway groups with order $4157776806543360000=2^{21}.3^9.5^4.7^2.11.13.23$ and has $22$ conjugacy classes of maximal subgroups. In this paper, we discuss a group of the form $\overline{G}=N\colon G$, where $N=2^{11}$ and $G=M_{24}$. This group $\overline{G}=N\colon G=2^{11}\colon M_{24}$ is a split extension of an elementary abelian group $N=2^{11}$ by a Mathieu group $G=M_{24}$. Using the computed Fischer matrices for each class representative $g$ of $G$ and ordinary character tables of the inertia factor groups of $G$, we obtain the full character table of $\overline{G}$. The complete fusion of $\overline{G}$ into its mother group $Co_1$ is also determined using the permutation character of $Co_1$.Keywords: Conway group, conjugacy classes, Fischer matrices, Fusions, Permutation character